spBayesSurv: Fitting Bayesian Spatial Survival Models Using R
Author ORCID Identifier
Haiming Zhou:https://orcid.org/0000-0002-2777-5354
Publication Title
Journal of Statistical Software
ISSN
15487660
E-ISSN
43888
Document Type
Article
Abstract
Spatial survival analysis has received a great deal of attention over the last 20 years due to the important role that geographical information can play in predicting survival. This paper provides an introduction to a set of programs for implementing some Bayesian spatial survival models in R using the package spBayesSurv. The function survregbayes includes the three most commonly-used semiparametric models: proportional hazards, proportional odds, and accelerated failure time. All manner of censored survival times are simultaneously accommodated including uncensored, interval censored, current-status, left and right censored, and mixtures of these. Left-truncated data are also accommodated. Time-dependent covariates are allowed under the piecewise constant assumption. Both georeferenced and areally observed spatial locations are handled via frailties. Model fit is assessed with conditional Cox-Snell residual plots, and model choice is carried out via the log pseudo marginal likelihood, the deviance information criterion and the Watanabe-Akaike information criterion. The accelerated failure time frailty model with a covariate-dependent baseline is included in the function frailtyGAFT. In addition, the package also provides two marginal survival models: proportional hazards and linear dependent Dirichlet process mixtures, where the spatial dependence is modeled via spatial copulas. Note that the package can also handle non-spatial data using non-spatial versions of the aforementioned models.
Publication Date
1-1-2020
DOI
10.18637/jss.v092.i09
Keywords
Bayesian nonparametric, Parametric models, Semiparametric models, Spatial dependence, Survival analysis
Recommended Citation
Zhou, Haiming; Hanson, Timothy; and Zhang, Jiajia, "spBayesSurv: Fitting Bayesian Spatial Survival Models Using R" (2020). NIU Bibliography. 468.
https://huskiecommons.lib.niu.edu/niubib/468
Department
Department of Statistics and Actuarial Science
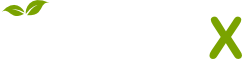
- Citations
- Policy Citations: 1
- Citation Indexes: 21
- Usage
- Abstract Views: 4
- Captures
- Readers: 74